Student News
CS Students Win Best Paper Honors
Students work alongside faculty as colleagues, investigating open-ended questions, producing world-class research, presenting findings at national conferences and, sometimes, winning honors for their work.
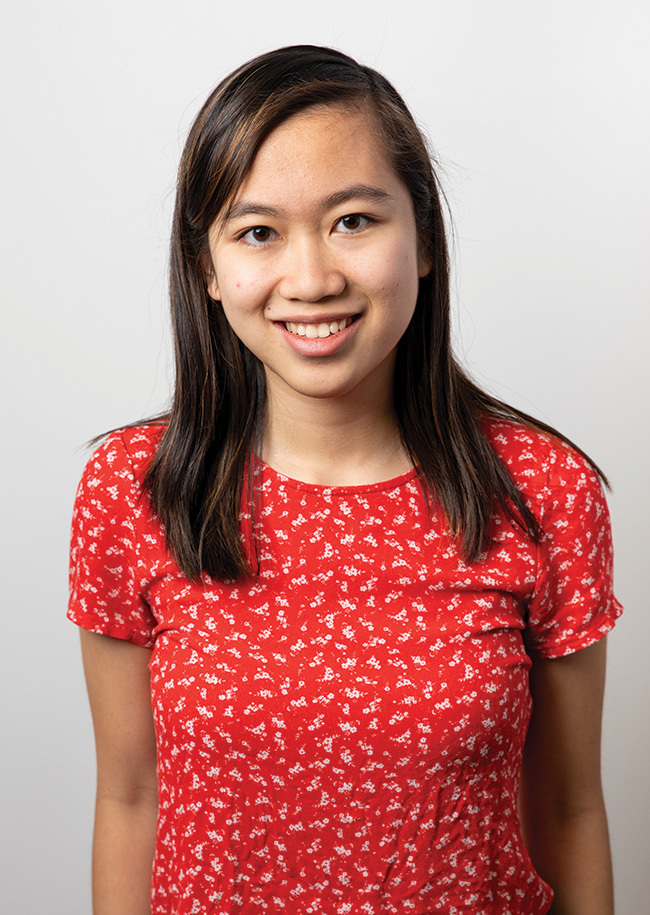
Student: An Nguyen ’22
Professor and co-author: Colleen Lewis
Organization: SIGCSE 2020 Technical Symposium
Paper: “Competitive Enrollment Policies in Computing Departments Negatively Predict First-Year Students’ Sense of Belonging, Self- Efficacy, and Perception of Department” (Award for Best Paper/CS education research track)
Nguyen explains: “To identify relationships between those policies and students’ experiences, we linked survey data from 1,245 first-year students in 80 CS departments to a dataset of department policies. We found that competitive enrollment negatively predicts first-year students’ perception of the computing department as welcoming, their sense of belonging and their self-efficacy in computing. Both belonging and self-efficacy are known predictors of student retention in CS. In addition, these relationships are stronger for students without pre-college computing experience. Our classification of institutions as competitive is conservative, and false positives are likely. This biases our results and suggests that the negative relationships we found are an underestimation of the effects of competitive enrollment.”
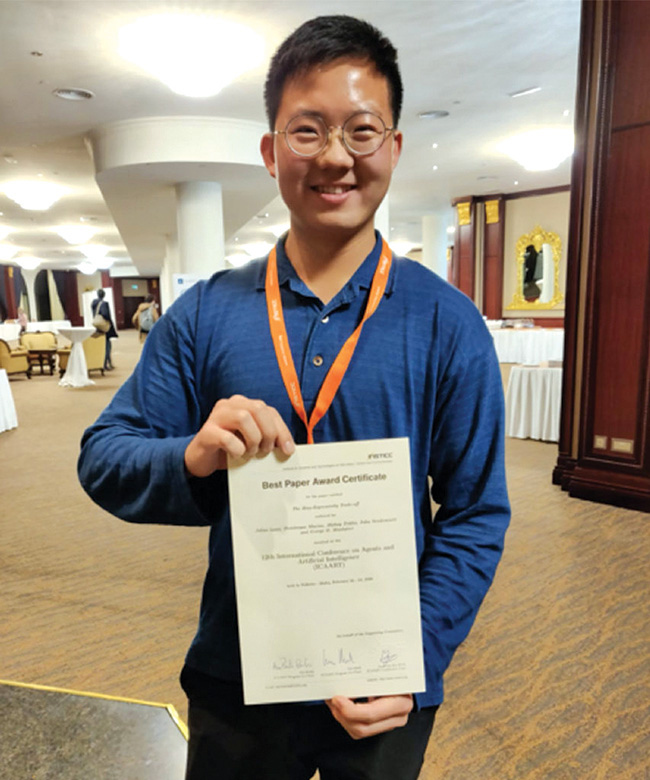
Students: Julius Lauw ’20, Dominique Macias ’19, Akshay Trikha ’21 and Julia Vendemiatti ’21 (computer science)
Professor and co-author: George Montañez Organization: 12th International Conference on Agents and Artificial Intelligence
Paper: “The Bias-Expressivity Trade-off” (Best Paper; ICAART 2020 had just a 16% acceptance rate for full papers. The Best Paper award is the first for Montañez’s research lab at Harvey Mudd and his fifth conference award overall.)
On behalf of the team, Lauw explains: “One of the strongest driving forces of this research is our team’s goal to formalize the theory of overfitting and underfitting in machine learning problems. The first step in achieving this target is to design a theoretical framework that allows us to derive bounds on the expressivity of machine learning algorithms. By justifying that there is an inherent trade-off between the expressivity and the amount of bias induced in a machine learning algorithm in this paper, we could potentially use this framework to estimate the expressivity of machine learning algorithms based on the amount of bias induced in the algorithm itself.”