Virtually Viral
Epidemiologists add simulation models, like the one created by Nadia Abuelezam ’09, to their arsenal in the fight to slow the spread of HIV.
The growing urgency to learn more about how epidemics spread among large populations has inspired epidemiologists to construct a world of their own—virtual populations of fictional citizens calibrated to mimic the traits of real people in a selected area.
Nadia Abuelezam ’09 is at work on the HIV Calibrated Dynamic Model (HIV-CDM), a simulation tool that uses sophisticated statistical methods to recreate, with startling accuracy, the dynamics of a pathogen on the move.
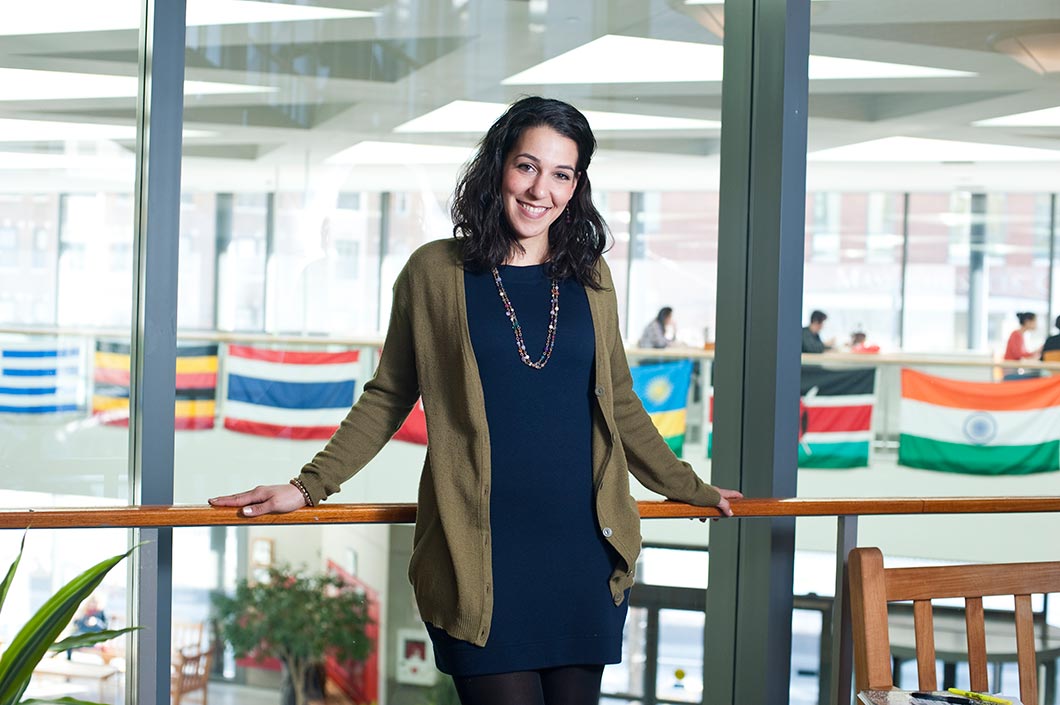
A joint mathematics and biology major, Abuelezam went on to study epidemiology at the Harvard T. H. Chan School of Public Health, where she earned her doctorate and is now a postdoctoral research fellow. “At Harvey Mudd, I got this rigorous quantitative education, and the math faculty members were spectacular. I was drawn into public health because I could apply the math and influence people’s lives.”
The HIV-CDM simulates reality in South Africa. “Each person in the simulation model has various characteristics: gender, sexual tendencies and health profiles. They interact with others in this simulated world. We have set up rules for behavior—for instance, someone with high-risk behavior would have multiple partners and likely one with HIV. When we run the model, it allows us to see the spread of HIV in the network, and we can also follow the health progression of individuals once they have HIV. We do everything from simulating initial transmission to simulating treatment and prevention on the far end.”
The broader benefits of models like the HIV-CDM become clear as one considers their operating scale and flexibility. “What’s unique about simulation models in an epidemiologic context is that they take data from other epidemiological studies and provide a framework for prediction and simulation that other methods don’t. We can test the impact of prevention methods or ask hypothetical questions. This is something that is difficult to do with observational data.”
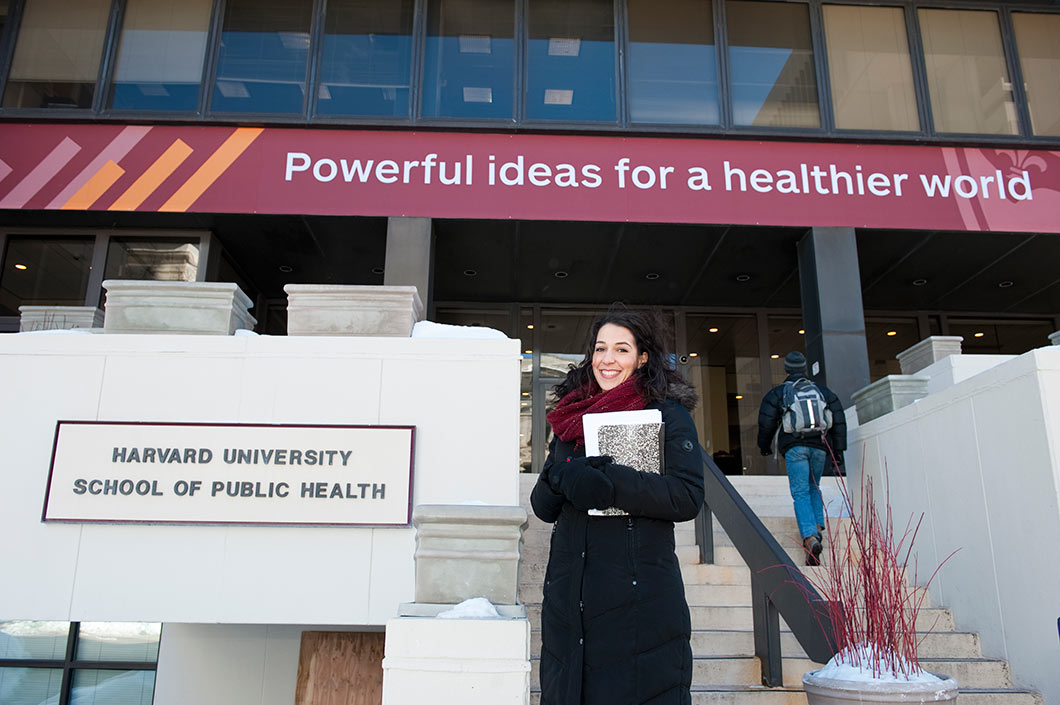
The model is protean in its complexity and beholden to the accuracy of the input data and the modeler’s assumptions. “This is something we struggle with,” Abuelezam acknowledges. “All the values for our parameters come from published reports on South Africa, and there are also assumptions we make. Some are stronger than others.”
Certain fundamental data points for the HIV-CDM relate to stigmatized human activities and conditions or other personal or social characteristics that are difficult to ascertain from traditional epidemiologic methods. Other times, information is simply not fully understood. A calibration technique known as Bayesian melding allows the model to bypass these apparent roadblocks.
In simple terms, Bayesian statistical analysis computes and takes into account comprehensively all the possibilities that could play a factor in a particular outcome to determine how likely that particular outcome is. Bayesian melding goes one step further. In the face of incomplete information and the added possibility of unforeseen events taking place within the system, the technique narrows the output range of a model and casts aside outliers.
Where data are less than robust, researchers assign credible ranges for given parameters, whether it be the potential number of sexual partners or the prevalence of HIV at a particular point in time. “These ranges form the upper and lower bounds for that parameter. We then examine the parameter space defined by those ranges to fit the model’s outcomes to existing data,” Abuelezam explains.
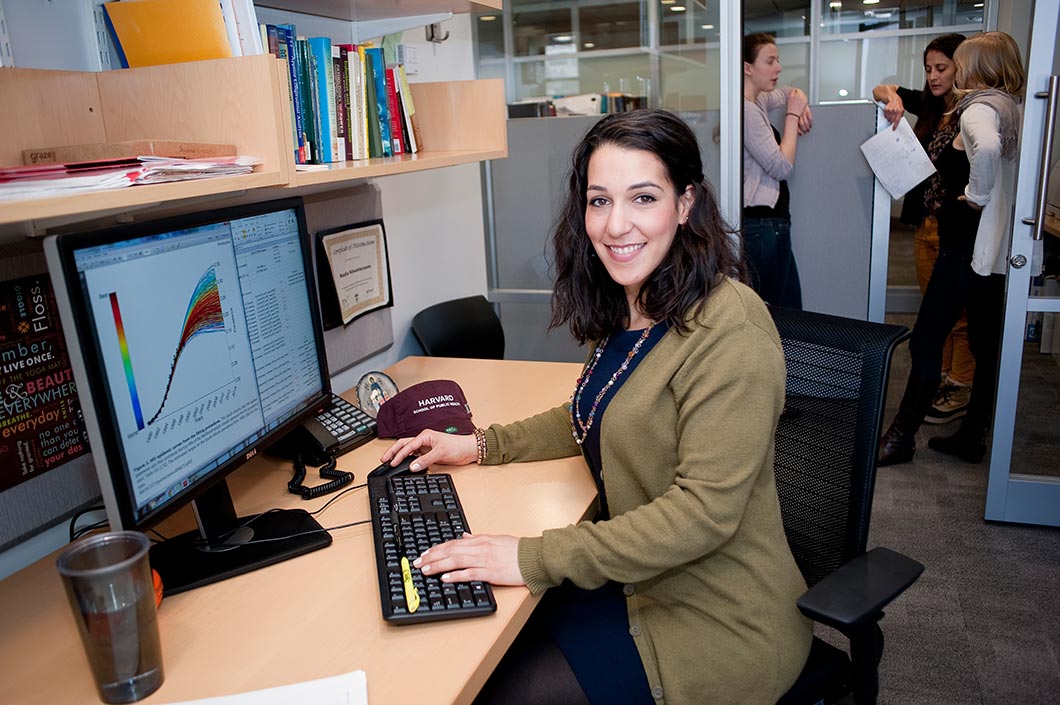
To complicate matters, the HIV-CDM is dealing with a dozen or more such parameters simultaneously. Think of each parameter as a slider on a sound-mixing console, for example. Using complex calculations and running the model thousands of times to determine the most prevalent results, you can adjust each slider until the final outputs of the model are in tune with the observed state of affairs of the population you are recreating. You then have an operational engine. To game out different containment strategies for a disease, try different inputs.
“That’s the next step,” Abuelezam says. “It’s great that we can simulate a population, but what can we say about the future? What will it take to eliminate HIV in South Africa? What combination of treatment and prevention programs is most effective? When we answer these questions, we can make policy recommendations to try to ensure people live long, healthy, uncomplicated lives.”
Epidemiology is two things. One is the analysis of a disease or social problem. The second part is taking what you have learned and trying to influence people’s behavior to improve health.
– Nadia Abuelezam ’09
With epidemics so much in the news, Abuelezam is used to fielding questions about them. “Of course, Ebola comes up frequently, and we have multiple teams here working on it. Simulation modeling is universal to epidemic disease, so Ebola is prime for such treatment, but it’s a different beast than HIV. It’s easier to acquire, for obvious reasons. We don’t have the same amount of historical data that we have for HIV, but what we do have is intense information about Ebola’s geospatial spread. People get treatment quickly, and so you can pinpoint with GPS places where people have it and subsequent cases that come up.”
The mention of the current resurgence of measles, a disease for which there is a safe and readily available vaccine, elicits polite exasperation from Abuelezam. “Epidemiology is two things. One is the analysis of a disease or social problem. The second part is taking what you have learned and trying to influence people’s behavior to improve health.”
A new project that Abuelezam is working on involves wading into social networks. “We want to learn more about hidden and at-risk populations in the Middle East and North Africa, where HIV transmission is on the rise. We plan to use existing social networking platforms to infer information about the sexual network in locations where traditional epidemiologic studies are impossible to conduct. It’s a very touchy project, and scary for a lot of people, because there is hostile legislation toward these groups in the region. Obviously, we don’t want to do anything that would put people in harm’s way. We do want to understand whether they are at risk and if they have access to prevention
and treatment.”
The Facts on HIV/AIDS
11.7 MILLION
At the end of 2013, 11.7 million people had access to antiretroviral therapy in low- and middle-income countries.
35 MILLION
At the end of 2013, 35 million people were living with HIV.
28 MILLION
Over 28 million people are eligible for antiretroviral therapy under WHO 2013 consolidated antiretroviral guidelines.
Source: who.int/hiv/en
Abuelezam has worked in Africa before and has been fascinated by it since she was a child. “The continent, the geography, but also the people in need.” She took a class on HIV at Harvey Mudd with Karl Haushalter, associate professor of chemistry and biology. Subsequently, through the class, she was able to arrange work in Uganda with The AIDS Support Organization. The trip was transformative.
“It was my first trip abroad. I had to learn to get myself where I needed to be, how to handle myself and communicate. It pushed me past a lot of my comfort points,” Abuelezam says. The trip had profound professional ramifications as well.
“There is no way I would be able to put what I am working on fully into context had I not gone. When you talk about age-disparate relations, rural areas with little access to health care, I saw all that firsthand. When I think about different aspects of the model, there is always a flashback in my mind:
You were there, you saw this happening. It’s improved my ability to model those situations
more accurately.”